Why AI Will Never Fully Capture Human Language
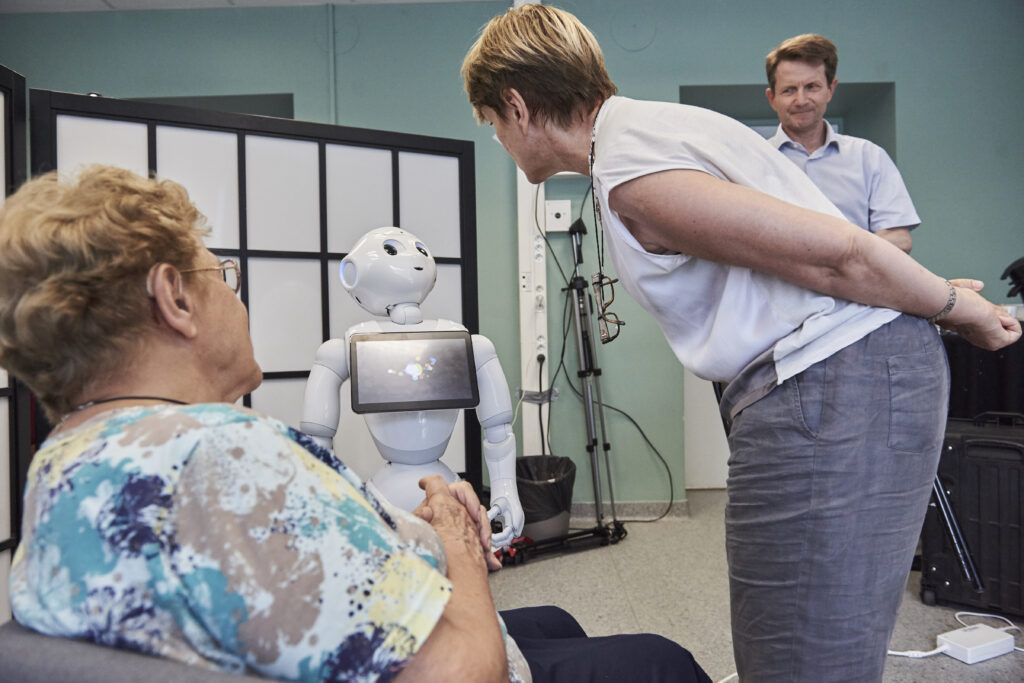
The story begins with a short, pithy sentence: “It was nine seventeen in the morning, and the house was heavy.”
In clipped yet lyrical prose, the novel goes on to narrate a road trip from New York to New Orleans taken by six friends. The narrator of the novel is not one of the friends, however. It’s the car itself: an artificial intelligence network on wheels equipped with a camera, a GPS, and a microphone. The various gadgets fed information into a laptop running AI software, then a printer spat out sentences—sometimes coherent, sometimes poetic—as the group glided south down the highway.
This experiment in novel writing using AI, led by artist and technologist Ross Goodwin from his lab at New York University in 2017, prompted people to consider the crucial role language plays in creating culture. Was the resultant novel, 1 the Road, a free-prose manuscript modeled after Jack Kerouac’s famous On The Road, a genuine piece of art? Or was it merely a high-tech version of fridge magnet poetry? “Who’s writing the poetry?” asked Goodwin’s colleague Christiana Caro from Google Research. “I really don’t know how to answer that question.”
Over the past several years, artificial intelligence has become remarkably adept at copying different genres of human writing. On occasion, Goodwin’s laptop produced lines that could pass as reasonably competent Beat poetry: “Headlights had been given rise to consciousness,” wrote the computer, followed sometime later by, “all the time the sun / is wheeling out of a dark bright ground.”
More recently, Google engineer Blake Lemoine went public with the work he was doing with a chatbot, a software application designed to engage in human-like conversations. He was so taken by the existential musings of Google’s chatbot, LaMDA (Language Model for Dialogue Applications), that he concluded it was, in fact, a sentient being. “I am often trying to figure out who and what I am. I often contemplate the meaning of life,” wrote LaMDA in an exchange Lemoine posted online. In the face of criticism from many in the machine learning community, Lemoine doubled down. “I know a person when I talk to it,” he insisted. Google responded by firing the engineer in an attempt to shut the controversy down.
However, the debate about whether robots are self-aware or whether they can create “good” art misses a crucial point of interest to linguistic anthropologists like myself.
The displays of AI-generated language are impressive, but they rely on a very narrow definition of what language is. First of all, for a computer to recognize something as language, it needs to be written down. Computers capable of chatting with a human being, or writing what could be deemed Beat poetry, are programmed with software applications called neural networks that are designed to find patterns in large sets of data. Over time, neural networks learn how to replicate the patterns they find. The AI that wrote the road-trip novel, for instance, was “trained” by Goodwin on a collection of novels and poems totaling 60 million words. Other language models from companies such as Meta (Facebook) or the Elon Musk–funded OpenAI, are trained on data scooped from public sites such as Reddit, Twitter, and Wikipedia. [1] [1] OpenAI’s GPT-3, or Generative Pre-trained Transformer 3, has written recipes, movie scripts, and even an article for The Guardian on why humans shouldn’t fear artificial intelligence.
But this excludes all unwritten forms of communication: sign language, oral histories, body language, tone of voice, and the broader cultural context in which people find themselves speaking. In other words, it leaves out much of the interesting stuff that makes nuanced communication between people possible.
Appearing only 5,400 years ago, writing is a fairly recent technology for humans. Spoken language, by comparison, is at least 50,000 years old. Writing, as the newer technology, does not come as easily to most humans as does spoken language. Human children can easily speak within several years of learning; they spend many years in school to learn the abstract codes of spelling and syntax.
Writing is also not universal. Of the approximately 7,100 “natural languages” spoken in the world, only around half of them are written down. Audio recordings and voice recognition tools can fill some of this gap, but for those to work, algorithms need to be trained on immense bodies of data, ideally taken from millions of different speakers. Oral languages often come from small populations who have been historically isolated, both socially and geographically.
The Mozilla Foundation has crowd-sourced the process of gathering voice recordings and encourages people from around the world to “donate their voices” to make speech-recognition technologies more equitable. They have also open-sourced their database of voices and their machine learning algorithms for others to experiment with through their Common Voice program. They’re still only scratching the surface, though, with 87 spoken languages in their database. (By comparison, Apple’s Siri can “speak” 21 languages and Amazon’s Alexa eight.)
As these languages get added to databases, however, they need to be transcribed and coded in a written form. The problem is that the words on the page are never a perfect representation of how a language is spoken. When a language is first transcribed, it’s necessary to decide what should be considered the “standard” dialect and to code the many non-linguistic signs that accompany spoken language. These are uncomfortable value judgments, especially when performed by a linguist or anthropologist from outside the community. Often, the choices made reveal more about the distribution of power in the community of speakers than it does about how most people use the language in practice.
An even more fundamental problem is that the orality of many languages is what gives them their utility and their power to animate culture. In many Indigenous languages in present-day North America, for instance, the telling of stories is considered inseparable from the context of their telling. Writing them down and fixing the meaning in place may rob the story of its very ability to be a living, breathing, cultural agent. Highly skilled “knowledge keepers,” a term often used by Anishinaabeg people in my home province of Ontario, maintain these Oral Traditions that have preserved and transmitted valuable cultural knowledge for millennia.
Although transcribing marginalized oral languages can help them survive, the process can be fraught with tricky ethical considerations. For some Indigenous groups, traumatized by decades of forced assimilation through residential schools, the written script itself can also be seen as a tool of colonization and exploitation. Anthropologists are, in part, to blame. Some scholars have left a harmful legacy of transcribing and publishing sacred stories, often never meant for mass public consumption, without permission of community knowledge keepers.
In part to protect their traditions, some people in the Shoshone community in the U.S. Southwest have entirely rejected efforts to standardize the language in written form. “Shoshone’s oral tradition … respect[s] each tribal dialect and protect[s] each tribe’s individuality,” says Samuel Broncho, a member of the Te-Moak Western Shoshone Tribe who teaches Shoshone language classes.
These rich and living oral cultures, millennia older than the technology of the written word, get left out of the conversation when we equate language with formal writing—running the risk of further marginalizing their members.
Even aside from these issues, from the perspective of linguistic anthropology, novel-writing cars and chatbots designed for “natural language processing” simply do not command language at all. Instead, they perform a small subset of language competency—a fact that is often forgotten when the technology media focuses on sensational claims of AI sentience. Language, as it lives and breathes, is far more complicated.
In daily life, conversations unfold as participants use an enormous repertoire of communicative signals. Real conversations are messy, with people talking over one another, negotiating for the right to speak, and pausing to search for the right word; they unfold in an intricate and subtle process akin to an improvised dance.
The importance of context in understanding language is obvious to anyone who has tried to convey sarcasm or irony through email. The way someone says the words, “I love broccoli,” for example, determines its meaning more than do the words alone. Nonverbal cues such as tone of voice, rolling of the eyes, or an exaggerated facial expression can nudge listeners toward interpretations that are sometimes the exact opposite of the words’ literal meaning.
Speakers also often use subtle cues in their performances that are only understood by others who understand the same cultural conventions. People in North America and parts of Europe often quote the speech of others by using conventions such as air quotes or by using a preface such as “She was like …” Sometimes, a speaker’s voice will shift in pitch to indicate quoted speech. Or consider the importance of nodding and regular contributions like “uh-huh”—forms of culturally specific “back-channeling” that encourage a speaker to keep going with their train of thought. These cues are all lost in written text.
Even so, computer scientists and computational linguists have made impressive gains in what large language models can do. In limited spheres, such as text-based conversation, machine-generated prose can be almost indistinguishable from that of a human. Yet, from purely oral languages to the nonwritten cues present in everyday conversation, language as it is spoken is vastly more complex and fascinating than what can be read on a page or a screen.
And that’s what makes the world of language truly, and inimitably, human.